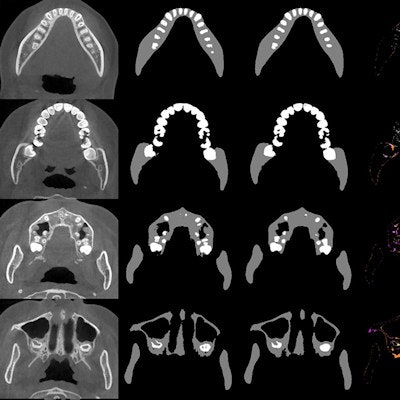
Researchers created an algorithm that shows the potential of artificial intelligence (AI) for dentistry. While it took humans nearly five hours to segment the teeth and jaw on a cone-beam computed tomography (CBCT) scan, an algorithm completed the task in just 25 seconds.
Despite the astounding time difference, the algorithm's segmentation showed high agreement with the segmentation done by four practicing dentists. The findings demonstrate that using a mixed-scale dense (MS-D) algorithm could one day make digital dentistry feasible for almost every patient.
"The MS-D network trained for multiclass segmentation would therefore make patient-specific orthodontic treatment more feasible by strongly reducing the time required to segment multiple anatomic structures in CBCT scans," wrote the authors, led by H. Wang, from the department of oral and maxillofacial surgery/oral pathology at Amsterdam UMC in the Netherlands (Journal of Dental Research, March 31, 2021).
Orthodontists employ the use of CBCT imaging to accurately assess complex malocclusions. Those same scans can be used to create 3D models for further diagnosis and treatment planning.
Currently, image segmentation is the most challenging part of converting a CBCT scan to a 3D model, the authors noted. The research focused on training a promising novel algorithm, the MS-D neural network, to simultaneously segment both the jaw and teeth from CBCT scans.
The team used 28 CBCT scans acquired from patients ages 11 to 24 who were set to undergo orthodontic treatment. Four dentists with at least two years of experience segmented the scans into three classes: teeth, jaw, and background.
The algorithm produced similar results to the segmentation done by the dentists, achieving a similarity index of 0.934 for distinguishing jaw and 0.945 for identifying teeth.
The results deviated from the dentists' by just 0.204 mm for teeth and 0.390 mm for jaw. It also took 25 seconds to segment both the jaw and teeth for one CBCT scan, "markedly reducing the time required for segmentation," the authors wrote.
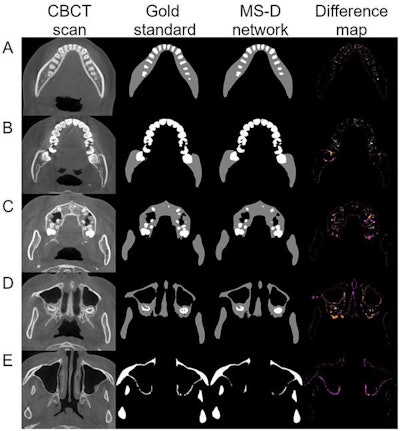
In one example, the difference map showed the algorithm erred mostly at the edges of segmentation with a difference of about 1 pixel. The algorithm also missed some thin bony structures around the maxillary sinus, the authors noted.
Importantly, none of the patients had fillings, implants, or crowns, so the scans were free of metal artifacts. The authors advised that future studies include patients with more complicated dental status.
"These results demonstrate that deep learning has the potential to accurately and simultaneously segment jaw and teeth in CBCT scans," the authors concluded. "This will substantially reduce the amount of time and effort spent in clinical settings, thereby facilitating patient-specific orthodontic treatment."